Is there any way for manufacturers to predict machine failures before they happen to avoid supply chain disruptions, delay and customer dissatisfaction? An emerging solution is the use of Artificial Intelligence (AI) data analytics in the supply chain, which has the most potential to minimize supply chain disruption as well as to dramatically reduce costs of supply chain.
Table of Contents
Predictive Analytics
According to Wollenhaupt (2016), downtime in auto manufacturing can cost $1.3 million per hour, according to published reports. The diagram below depicts the prediction of a motor failure.
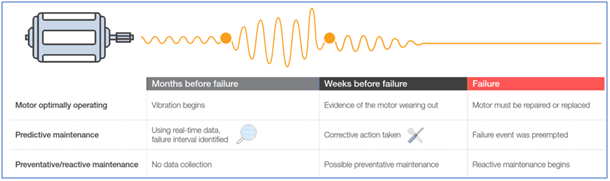
Manufacturers now have the tools and resources to apply “predictive maintenance” on their machines and factories. With predictive maintenance, significant reductions in unplanned downtime can save millions of dollars and keep customers happy.
The Data Analytics Eco-System to Prevent Downtime
Manufacturers can now use AI technology, Machine Learning, Deep Learning, IoT and Big Data to integrate smart sensors with their machinery and to develop smarter supply chains and manufacturing processes. Doing so will increase visibility into their supply chains and a greater ability to reduce supply chain disruptions through proactive and predictive maintenance.
1. Artificial Intelligence (AI)
Artificial intelligence is any computer program that does something smart. It can be a stack of a complex statistical model or if-then statements. AI can refer to anything from a computer program playing chess, to a voice-recognition system like Alexa. The models for AI technology take a lot of insight to deliver and can only be achieved through proper analysis and data gathering.
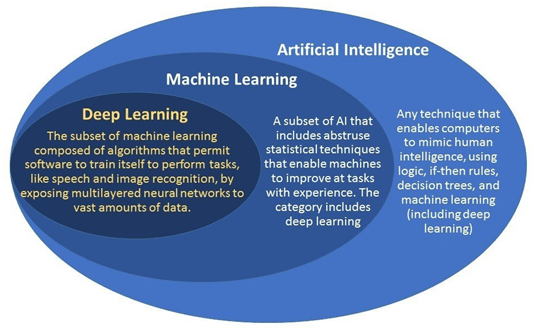
2. Machine Learning (ML)
Machine learning is a subset of AI. The theory is simple, machines take data and ‘learn’ for themselves. It is currently the most promising tool in the AI pool for businesses. Machine learning systems can quickly apply knowledge and training from large datasets to excel at facial recognition, speech recognition, object recognition, translation, and many other tasks.
3. Deep Learning (DL)
Deep learning is a subset of ML. Deep artificial neural networks are a set of algorithms reaching new levels of accuracy for many important problems, such as image recognition, sound recognition, recommender systems, etc.It uses some machine learning techniques to solve real-world problems by tapping into neural networks that simulate human decision-making. For example, a deep learning algorithm could be trained to ‘learn’ how a dog looks like. It would take an enormous dataset of images for it to understand the minor details that distinguish a dog from a wolf or a fox.
4. Internet of Things (IoT)
IoT is the outcome of technology advances in four main areas:
(1) Connected devices and sensors. Manufacturers are building sophisticated, connected gateway products. These products provide standardized ways to talk to the world of sensors.
(2) Ubiquitous data networks. Telecom companies are building better and cheaper data networks with widespread coverage.
(3) The rise of the cloud and the big shift from enterprise to Software as a Service (SaaS) platforms.
(4) Big-Data Technology: The ability to process large amounts of data in a standardized way.
This means that every ‘thing’ around you can connect and communicate its status back to software platforms. Cloud-based software platforms built on latest advances in big data technology can swiftly process this information and offer insights- a direct prerequisite for Predictive Maintenance.
5. Big Data
We generate data whenever we go online, when we carry our GPS-equipped smartphones, when we communicate with our friends through social media or chat applications, and when we shop. You could say we leave digital footprints with everything we do that involves a digital action, which is almost everything.
On top of this, the amount of machine-generated data is rapidly growing too. Data is generated and shared when our “smart” home devices communicate with each other or with their home servers. Industrial machinery in plants and factories around the world are increasingly equipped with sensors that gather and transmit data.The term “Big Data” refers to the collection of all this data and our ability to use it to our advantage across a wide range of areas, including business.
The Interdependence of AI and Big Data
AI is essential to the next wave of “Big Data” analytics. It is a vital tool for achieving a higher maturity and scale in data analytics, and for allowing broader deployment of advanced analytics.
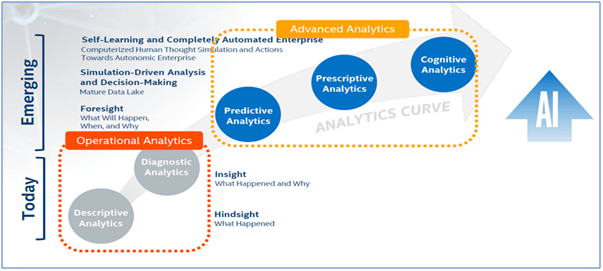
As shown in the chart above, there are three types of advanced analytics technologies. The more basic, and most widely used, is predictive analytics which employs technologies such as statistical modeling and simulation. Like “Predictive analytics” looks at the temperature profile and tells you it is likely to fail in X amount of time.
The next step up is prescriptive analytics which employs optimization, heuristics and rules-based “expert systems” with business rules defined by humans to solve a supply chain problem. Like “Prescriptive analytics” tells you that if you slow the equipment down by Y%, the time to failure can be doubled, putting you within the already scheduled maintenance window and revealing whether you can still meet planned production requirements.”
Artificial intelligence is the most leading-edge form of advanced analytics which includes machine learning, deep learning, natural language processing and “cognitive advisers” which are AI-based solutions that interact with business users through natural language.AI has opened new horizons in the industrial sector from self-adaptive manufacturing to predictive maintenance, automatic quality control and driverless vehicles.
Availability of Big Data
In 2020, the digital universe is expected to reach 44 Zettabytes (1 Zettabyte is equal to 1 Billion Terabytes). Data valuable for enterprises, especially unstructured data from IoT devices and non-traditional sources, is projected to increase both in absolute and relative sizes.
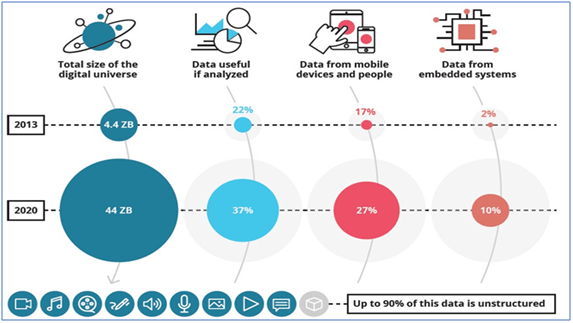
Managing the “Flood of Data”
While the cloud is a good place to store data and train machine learning models, it is often unsuitable for real-time data collection or analysis. Bandwidth is a particular challenge, as industrial environments typically lack the network capacity to ship all sensor data up to the cloud. Thus, cloud-based analytics are limited to a batch or micro-batch analysis, making it easy to miss blips in the numbers.
Edge computing facilitates the processing of delay-sensitive and bandwidth-hungry applications near the data source. Most of it will be processed locally by specialized “Edge” computing devices. Edge computing is expected act as a strategic brain behind IoT. Edge computing is utilized to reduce the amount of data sent to the cloud and decrease service access latency.
The full content is only visible to SIPMM members
Already a member? Please Login to continue reading.
References
Abdul Kareem Mohamed Yasin, GDSCM. (2018). “AI Technologies Enhancing Supply Chain Management”. Retrieved from SIPMM: https://publication.sipmm.edu.sg/ai-technologies-enhancing-supply-chain-management/, accessed 12/12/2018.
Meenal Dhande. (2017). “What is the difference between AI, machine learning and deep learning”. Retrieved from https://www.geospatialworld.net/blogs/difference-between-ai%EF%BB%BF-machine-learning-and-deep-learning, accessed 12/12/2018.
Pierce Lamb. (2018). “IoT Analytics for Predictive Maintenance”. Retrieved from https://www.snappydata.io/solutions/iot-analytics-predictive-maintenance, accessed 12/12/2018.
Sylvia Lee Shui Cha, GDPM. (2017). “How Artificial Intelligence Can Revolutionize Procurement”. Retrieved from SIPMM: https://publication.sipmm.edu.sg/how-artificial-intelligence-revolutionize-procurement/, accessed 12/12/2018.
Wollenhaupt, G. (2016). “IoT Slashes Downtime with Predictive Maintenance”. Retrieved from https://www.ptc.com/en/product-lifecycle-report/iot-slashes-downtime-with-predictive-maintenance, accessed 15/12/2018.